Radio Cloud Native – Week of May 11th, 2022
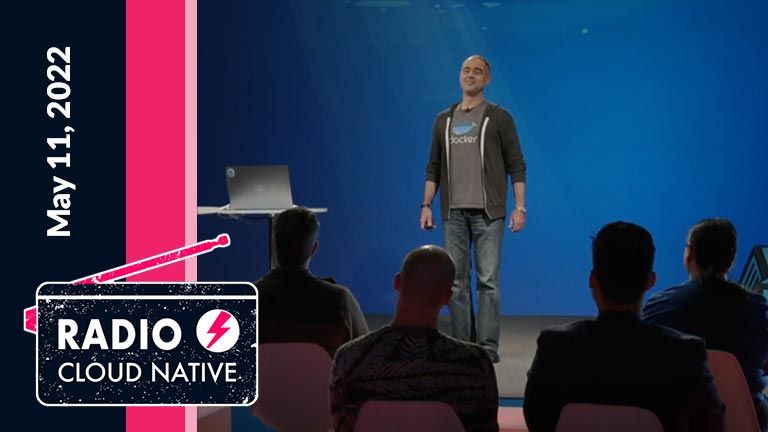
Every Wednesday, Nick Chase and Eric Gregory from Mirantis go over the week’s cloud native and industry news.
This week they discussed:
Artificial Intelligence shows signs that it’s reaching the common person
NIST changes
Palantir is back, and it’s got a Blanket Purchase Agreement at the Department of Health and Human Services
IBM on quantum scaling
You can watch the full replay below:
To join Nick and Eric next Wednesday, May 18, at 1:00pm EST/10:00am PST, follow Mirantis on LinkedIn to receive our announcement of next week’s topics.
Docker Extensions
Eric Gregory: Hi everyone, and welcome to Radio Cloud Native from Mirantis. Every week, we break down tech news in the cloud native world and beyond. I’m Eric Gregory—
Nick Chase: and I’m Nick Chase. This week, we’ll be talking about news from DockerCon, developments at Google Cloud, the latest in AI and quantum computing, and more.
Eric Gregory: DockerCon started this week, and it’s brought some significant announcements from Docker Inc. First off, they announced the beta release of a new extensions feature for Docker Desktop, along with a Docker Extensions SDK for creating new add-ons. This is one of those “Surprise! It’s available” announcements, so you can update Docker Desktop and have a look right now–the feature is available at personal and paid tiers alike.
Docker themselves developed some of the first few extensions to demonstrate the concept, adding extensions for exploring container logs and managing disk space used by Docker.
Docker Inc also announced that a Linux version of Docker Desktop is now generally available, bringing a unified experience across Windows, Mac, and Linux. Some listeners’ first thought is probably going to be, “What about Docker Engine?” and that remains available. Right now, Docker Desktop for Linux is available via deb and rpm packages with support for Ubuntu, Debian, and Fedora. For the tinkerers out there, they also say that they expect to add support for 64-bit Raspberry Pis over the next few weeks.
Source: Container Journal
Artificial Intelligence shows signs that it’s reaching the common person
Nick Chase: Artificial Intelligence always seems like this thing that you have to have a PhD to use, but we’ve got several stories this week about companies that are starting to move it into the realm of public usage.
In a blog post this week Cisco talked about predictive networks,e explaining in actually some fairly human-friendly terms how machine learning works and talking about how this new this new network could be used to not only predict when network errors would happen, but also to remediate issues before they happen. Sound familiar, Eric?
Yes, so it seems that AIOps is finally inching its way towards reality. I should note that this is still pretty early days here, they’re only talking about predictive networks in vague terms and saying that they’ll be part of Cisco products at some point, which I completely applaud, but I’d love to see something more concrete.
Intel is also talking about a new AI/ML product, in this case focused on making it easier to do computer vision projects. The Register reports that “Intel is pitching Sonoma Creek as an “end-to-end AI development platform” that simplifies computer-vision model training for subject matter experts who don’t have data science experience.”
The software makes use of Intel’s open source OpenVINO toolkit, which does computer vision. And that’s useful not just in terms of recognizing things like who’s at your door, but also for use cases such as analyzing X-Rays, and so on.
One nice thing about Sonoma Creek is that it lets users improve the accuracy of the model. So for example if it were to misidentify a particular image, you can add additional images to the dataset, level them correctly, and then re-export the model. Kind of like the kids game. Did you ever play that?
Alibaba has open sourced the code for Federated Scope, which is a federated tool for machine learning. And this is kind of interesting, because they’re touting it as helping to provide privacy. Here’s why.
Normally, in order to train a model, you of course need to have a large data set. we’ve talked about that on multiple occasions. But how can you get that large data set without combining everyone’s private data together? Well the answer, it seems is to train locally, then send the results on to be combined with the results of everyone else.
It’s like the MapReduce algorithm, where you can process multiple datasets in parallel, then combine the results.
And it’s important that these models and tools are getting shared, because trying to do this yourself for anything of any size can require a ridiculous amount of resources. I’ll give you an example. This week Facebook’s parent company, Meta, shared the Open Pretrained Transformer, a giant language model, with academics. The full version of this model, OPT-175, has 175 billion parameters, and took 992 Nvidia 80GB A100 GPUs to train, and according to The Register it still took 35 attempts over 2 months. But they’re providing everything researchers need to run this model on only 16 Nvidia V100 GPUs.
And the reason that they’re offering this model to researchers is that these tools can be used to generate pretty convincing text, especially for generic things like sports scores, and so on, but like everything else AI related you’re often getting results that are biased or inaccurate, which, of course they are.
If you’re a researcher you can apply for access to this model, but if you’re not, they’re also providing access to the dataset they trained it on, as well as a smaller subset with only 66 Billion parameters.
Google Cloud TPU VMs reach general availability
Eric Gregory: Well, speaking of expanding access to machine learning and AI capabilities, Google Cloud announced that VMs for Tensor Processing Units (or TPUs) have reached general availability. Now, TPUs are application-specific integrated circuits developed by Google for neural network machine learning, and particularly for Google’s TensorFlow AI and machine learning library.
Cloud TPU VMs were first introduced last year in order to give users direct access to TPU host machines. Now Google claims the GA release brings greater optimization for large-scale recommendation and ranking workloads.
So, in addition to AI, Google Cloud is making some moves in the edge space, right?
Source: Google Cloud blog
Google buys MobileX, folds into Google Cloud
Nick Chase: According to TelecomTV, Google has bought the company originally set up by Deutsche Telecom to handle MobileX, an attempt to create a standard middleware lawyer for edge computing. The idea was that MobileX would provide a way for “federation between any standards-based mobile edge computing platform.” And in fact MobileX did have some success, an earlier this year, they were able to interconnect the Bridge Alliance Federated Edge Hub (FEH) and the MobiledgeX Edge-Cloud platform, for a successful interconnection of two multi-access edge computing (MEC) platforms, so that’s cool.
They also had deals with something like 26 different carriers, but what they didn’t have was prospects. Many of the major telcos are starting to standardize on various public cloud platforms, which probably played a big role in which Google felt like they needed to get their own. Google has already folded MobileX into Google Cloud, but it will be open sourcing the software, so it’s not all about control. Maybe just mostly, but not all.
Probably the closest analog you can get to this is Android, in which Google has open sourced the software, but largely controls it, and gets a portion of the take when developers make money in the Android Play store. Presumably the idea is tath they’ll be creating some sort of Edge application store and work it the same way.